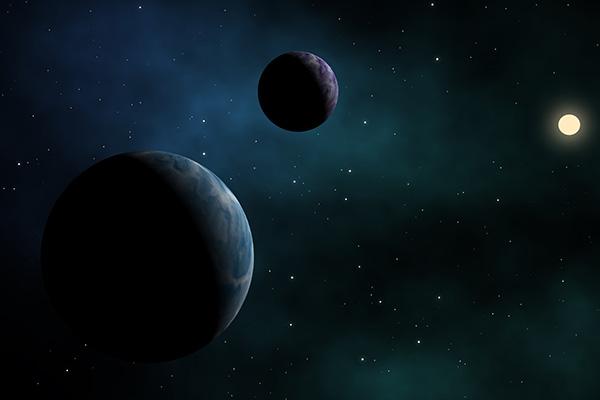
Title: Unlocking the Detection of Earth-Like Exoplanets by Mitigating Stellar Activity with Deep Learning
Speaker: Yinan Zhou (UT Austin)
Abstract:
Stellar activity remains the primary obstacle to detecting Earth-like planets via radial velocity (RV) measurements around solar-type stars. In this talk, I will introduce a deep learning-based algorithm designed to improve our understanding and mitigation of stellar activity by efficiently modelling it in the spectral shell domain, thereby enhancing the detection of Earth-like planets. This algorithm has been tested on both simulated spectra and real observations of three well-studied stars: Alpha Centauri B, Tau Ceti, and the Sun. By injecting simulated planetary signals at the spectral level, we demonstrate that our algorithm can achieve, for Alpha Centauri B and Tau Ceti, a detection threshold of 0.5 m/s in semi-amplitude for planets with periods ranging from 10 to 300 days. On the HARPS-N solar dataset, our algorithm is even more efficient at mitigating stellar activity signals and can reach a threshold of 0.2 m/s, which would correspond to a 2.2 Earth-mass planet on the orbit of the Earth. This is the first time that such low detection thresholds have been reported for the Sun, as well as for other stars, and therefore highlights the efficiency of our deep learning-based algorithm in mitigating stellar activity in RV measurements. To further characterize the model's robustness across different stellar types, we also evaluate its ability to model the flux effect and the inhibition of convective blueshift, using SOAP-GPU simulated solar spectra based on SDO/HMI images. These results highlight the promise of deep learning for advancing RV planet detection into the sub-Earth mass regime.